The following is the established format for referencing this article:
Schoen, J. E., S. M. Miller, M. W. Reudink, Q. Jennings, and S. M. Mahoney. 2023. Disentangling the mechanisms of signal evolution in Tyrannidae flycatchers, part I: song is constrained by morphology and covaries with ecological factors. Journal of Field Ornithology 94(3):6.ABSTRACT
Acoustic signals mediate key animal interactions and can evolve through a variety of factors. Signal divergence can reinforce pre-zygotic barriers and minimize costly hybridizations among closely related species or partition acoustic space to avoid signal interference. To unravel the drivers of song evolution, it is critical to simultaneously test multiple evolutionary axes leading to heterospecific song variation (e.g., the role of morphology, ecology, and heterospecific recognition). Tyrannidae is the largest Passeriformes family and occurs across a broad environmental gradient. Tyrannids are suboscines, so song variation represents evolutionary differences that are not confounded by social learning. Several genera show conserved plumage coloration, but exhibit pronounced vocal differences. In the first of our two-part paper on signal ecology and evolution, we leveraged a large-scale song dataset to unravel song divergence in Tyrannidae (n = 282 species) by testing the relative influence of morphology (body and bill size), ecology (vegetation cover, diet, climate), and heterospecific proximity on song evolution. Based on phylogenetically controlled analyses, we found Tyrannidae song evolution was primarily driven by morphological adaptation, where larger-bodied birds with heftier bills sang lower frequency and slower paced songs. Pairwise song differences were weakly related to heterospecific proximity of some genera, lending support to the species recognition hypothesis or drift. Given that many flycatchers are habitat specialists, natural selection acting on bill morphology and body size in specific environmental-contexts may shape song among tyrannids. By simultaneously testing the relative roles of morphological, ecological, and geographical factors on song evolution, our study highlights the complexity of suboscine song evolution and the importance of large-scale comparative studies that test multiple evolutionary hypotheses.
RESUMEN
Las señales acústicas median interacciones animales clave y pueden evolucionar a través de una variedad de factores. La divergencia en la señal puede reforzar barreras pre-cigóticas y minimizar hibridación costosa entre especies cercanamente emparentadas o particionar el espacio acústico para evitar interferencia en la señal. Para desenredar los determinantes de la evolución del canto, es crítico evaluar múltiples ejes evolutivos simultáneamente que llevan a la variación hetero específica en el canto (e.g., el rol de la morfología, ecología y reconocimiento heteroespecífico). La familia Tyrannidae es la más grande de los Passeriformes y ocurre a lo largo de un amplio gradiente ambiental. Los tiranidos son suboscinos, por lo que la variación en el canto representa diferencias evolutivas que no están confundidas con el aprendizaje social. En la primera de las dos partes de nuestro estudio sobre la ecología y evolución de las señales, apalancamos una base de datos de gran escala para desenredar la divergencia en el canto en la familia Tyrannidae (n = 282 especies) evaluando la influencia relativa de la morfología (tamaño corporal y del pico), ecología (cobertura de la vegetación, dieta y clima) y proximidad hetero específica sobre la evolución del canto. Basado en análisis controlados filogenéticamente, encontramos que la evolución del canto en la familia Tyrannidae esta principalmente determinada por la adaptación morfológica, donde especies con tamaños corporales mas grandes y picos más pesados cantan a frecuencias más bajas y a un ritmo más lento. Diferencias pareadas en el canto estuvieron débilmente relacionadas con la proximidad hetero específica de algunos géneros, dando soporte a la hipótesis de reconocimiento interespecífico o deriva. Dado que muchos atrapamoscas son especialistas de hábitat, la selección natural que actúa sobre la morfología del pico y el tamaño corporal en contextos específicos al ambiente pueden moldear el canto en los tiránidos. A través de la evaluación simultánea de los roles relativos de factores morfológicos, ecológicos y geográficos sobre la evolución del canto, nuestro estudio resalta la complejidad de la evolución del canto en suboscinos y la importancia de estudios comparativos de gran escala para evaluar múltiples hipótesis evolutivas.
INTRODUCTION
Birds produce vocalizations that mediate key behaviors (Bradbury and Vehrencamp 1998). Songs can diverge through natural selection, sexual selection, as a result of neutral processes like genetic drift (Dynesius and Jansson 2000), arbitrary mate choice (Prum 2010), or a combination of these phenomena. When songs among populations differ to such an extent that individuals no longer recognize each other as conspecifics, species borders become established and song can function as a pre-mating isolating mechanism (Bradbury and Vehrencamp 1998, Seddon 2005, Mason and Burns 2015, Derryberry et al. 2018). Understanding the ways in which differences in song between related species arise and are maintained by selection is an important topic in evolutionary ecology because it is key to understanding speciation (Mayr 1942, Seddon 2005).
Environmental factors can influence song structure due to differences in the sound transmission properties of habitats through sensory drive or acoustic adaptation (Morton 1975, Endler et al. 1980). Sounds of differing frequency and temporal elements do not propagate similarly in all habitats, so selection should favor songs that optimize transmission given a species’ environmental context (Morton 1975). For example, acoustic signals in forests are subject to scattering by spherical spreading, refraction, reflection, or diffraction from vegetation (Wiley and Richards 1978, Richards and Wiley 1980, Derryberry et al. 2018). Consequently, songs with repeated short notes are found in open habitats and songs in forested habitats are longer in duration and lower in frequency (Wiley 1991, Richards and Wiley 1980, Nicholls and Goldizen 2006).
The acoustic adaptation hypothesis, however, is not supported in all passeriforms. Recent studies on song frequency across over 5000 passerine species found that although there is evidence supporting a relationship between song frequency and body mass, there is no generalized relationship between song frequency and habitat (Mikula et al. 2020). These results suggest that variation in passerine song frequency may be primarily driven by selection for morphology, rather than habitat-related selection on sound (Mikula et al. 2020, Francis and Wilkins 2021); however, recent evidence supports sensory drive within species (Sebastianelli et al. 2022). Part of the reason for the mixed results of sensory drive is that previous studies are unclear on what portion of the hypothesis they are testing (Cummings and Endler 2018). The sensory drive model, modified from Endler (1992), can be divided into two components: sensory and signaling (Cummings and Endler 2018). The sensory component of the model predicts a strong correlation between receiver sensory detection properties (e.g., sensory system, brain characteristics, and perception of signals) with features within the physical environment (Cummings and Endler 2018). The signaling component may therefore evolve to optimize transmission given the physical and biotic environment (Cummings and Endler 2018).
Both ecology and morphology can also influence song structure (Derryberry et al. 2018). Morphological adaptation typically results when structural change in an organism provides it with either higher reproductive success or with greater survival. For example, the bill, which is typically under strong selection in the context of diet and foraging, is used in coordination with syrinx movements to modify sound (Derryberry et al. 2018). Indeed, variation in bill morphology driven by dietary access has been directly linked to variation in song structure and the song performance capabilities of birds (Podos 2001, Grant and Grant 2006). Morphological selection for bill size therefore represents a potential driver of song divergence (Wilkins et al. 2013). In addition to changes in bill size, body size can also affect song structure; many larger-bodied species with larger bills produce lower frequency and slower paced sounds (Podos 2001).
Conspecific recognition is another driver of divergence in mating signals; signaling is predicted to differ between closely related species when their respective geographic ranges overlap (Simpson et al. 2021). If congeners co-habit the same environment, their song may become divergent to differentiate species and prevent costly interbreeding (Simpson et al. 2021). In contrast, allopatric species do not face the same pressures to differentiate their song because they are geographically isolated from their closely related species, but vocal differences between allopatric species can arise from neutral processes like genetic drift (Irwin et al. 2001). An alternative explanation to species recognition is that acoustic partitioning prevents signal interference with heterospecifics (Luther 2009).
Passeriformes accounts for over half of all extant bird species (Clayton et al. 2009). Within this order, the radiation of oscine (Suborder Passeri), and suboscine (Suborder Tyranni) birds began about 65 million years ago (Clayton et al. 2009). Tyrannidae is considered the largest family of passerine birds and its species breed throughout North and South America across broad climatic and ecological ranges (e.g., Johnson and Cicero 2002, Fig. 1A). Among species, Tyrannidae songs are highly variable (Fig. 1B-C). Tyrannids are suboscines, meaning their song development is innate and requires relatively less learning (reviewed in Francis et al. 2010, Liu et al. 2013). Song variation among suboscine species therefore represents genetic differences associated with reproductive barriers making Tyrannidae a useful group to investigate the ecological and morphological mechanisms that contribute to song variation because heterospecific differences are less susceptible to cultural evolution (Tobias et al. 2012).
The radiation of tyrannids is unique because many genera exhibit conservative plumage evolution with sister species that are nearly indistinguishable by plumage (e.g., Rheindt et al. 2008). Though some Tyrannidae sister species are morphologically similar, they can be distinguished by their vocalizations (Johnson and Cicero 2002, Rheindt et al. 2008). Additionally, sibling species often occur in distinctive habitats across broad geographic regions suggesting strong ecological selection may indirectly affect signal evolution (Johnson and Cicero 2002). Although several tyrannid genera are phenotypically similar but divergent in song and ecology (e.g., Cicero and Johnson 2002, Rheindt et al. 2008), others exhibit ornate plumage calling into question the relative importance of song or plumage in the radiation of Tyrannidae. In the first of a two-part paper on Tyrannidae signal evolution, we focus on how morphological, ecological, and geographic factors affect song evolution to assess how song evolution contributed to the radiation within the Family Tyrannidae (see Part II for our similar investigation into Tyrannidae plumage color evolution). To do this, we used phylogenetically controlled analyses to assess the relative influence of morphology (body and bill size), ecology (vegetation cover, diet, and climate), and proximity to heterospecifics to test the following hypotheses: (1) the signaling component of the sensory drive hypothesis, predicting acoustic signals covary with environmental factors that affect acoustic transmission; (2) the morphological adaptation hypothesis, predicting larger body and bill morphology produces lower frequency and slower-paced songs; and (3) the species recognition hypothesis, which predicts congeners living in closer proximity will exhibit more pronounced song differences to minimize costly hybridization events. Whereas previous work tests single aspects of song evolution, we aimed to simultaneously test morphological, ecological, and geographical factors in song evolution. We additionally sought to broaden the field of avian ecology and evolution in historically underrepresented regions because many of the species in our study occur exclusively in Central and South America (Nuñez et al. 2021).
METHODS
Tyrannidae song recordings
The Family Tyrannidae comprises 399 species in 101 genera, living in a diversity of habitats across North and South America (Winkler et al. 2020). Many of the genera in the family have diversified into morphologically similar species with pronounced vocal differences. To assess song variation among species, we downloaded publicly available Tyrannidae songs from the Cornell Lab of Ornithology’s Macaulay Library (https://www.birds.cornell.edu/home/) and from https://xeno-canto.org. Only songs with a high signal-to-noise ratio (i.e., “A” quality from xeno-canto.org) were included in our dataset. “Song” was defined as vocalizations that exhibited tonal elements and were preceded and followed by intervals greater than one second (Demery et al. 2021). We did not set minimum duration requirements because Tyrannidae songs can be relatively simple, and some species exhibit a single tonal note (e.g., Fig. 1C and Cnemarchus erythropygius song is one note and 0.45 seconds in duration). Xeno-canto and the Macaulay library display song recordings on range maps, so to capture intraspecific geographic song variation, we sampled a maximum of five songs species-1 from across the species’ range whenever possible (Mason and Burns 2015). We assumed recordings from different locations and dates were from different individuals and we used recordings from males whenever possible to avoid intrasexual variation (Mason and Burns 2015). Although this approach is consistent with similar studies of song ecology and evolution (e.g., Mason and Burns 2015, Demery et al. 2021), it is possible that sex was misidentified by the recordist. A growing amount of evidence shows female song is prominent in birds (Riebel et al. 2019), and although our dataset did not allow us to test for sex differences, sex misidentification and sex differences in our data should diminish statistical effects. We recognize a large-scale test of sex differences in vocal evolution is needed and urge future studies to do so (Odom and Benedict 2018). To avoid recordings from non-breeding and/or migrating birds, only songs that provided specific location metadata and were recorded during the breeding season, i.e., May-July for Neotropical migrants and February-April for Central and South American residents (Freeman et al. 2017) were included. Migratory classification was determined from Tobias et al. (2022). Songs for 117 species were unavailable, or missing all or some of the above metadata, and were not included in our study. Our resulting dataset was 282 species from 85 genera (970 total songs, mean: 3.44 songs species-1, range: 1-5 songs species-1; Appendix 1).
Quantifying song structure characteristics
Consistent with previous work, we quantified song characteristics on one song per individual to avoid pseudoreplication (Mason and Burns 2015, Demery et al. 2021). We first digitized the recordings in Raven Pro (Cornell Lab of Ornithology) using the Hann window (size = 256 samples, 50% window overlap, DFT = 256 samples) with unsmoothed view and applied a high and low pass filter (1 kHz and 7 kHz, respectively). We then determined the beginning and end of the song (timestamp in seconds) and the number of notes. We did not quantify internote intervals because some species sing single-note songs. These data were then used in the sound analysis R package “warbleR” (Araya-Salas and Smith-Vidaurre 2017). We used the “spectro_analysis” command to automatically quantify frequency and temporal characteristics of each song (Appendix 2). We then calculated the mean value for each frequency and temporal measurement, for each species. To control for collinearity among song variables, we summarized the temporal and frequency measurement for each song using a phylogenetic principal components analysis (PPCA) in the “phytools” package for R (Revell 2012). To help with interpretation of the axis loadings, we multiplied PPCA values (song PC1 and 2) by -1 (Vehrencamp et al. 2003).
Ecology and morphology data collection
To examine the relationship between ecology, morphology, and song characteristics, we gathered bioclimatic variables and morphological estimates (Derryberry et al. 2018). Using WorldClim 2 (Fick and Hijmans 2017), we used annual mean temperature (BIO1, ºC) and annual precipitation (BIO12, mm; Appendix 1) because these variables may influence song transmission via signal overlap or alter sound propagation. To examine the relationship between song characteristics and morphology, we gathered bill morphology data from Pigot et al. (2020) and Tobias et al. (2022) and body mass data from Wilman et al. (2014). Bill morphology included nares bill length (mm), bill width (mm), and bill depth (mm) and body morphology included tarsus length (mm), wing length (mm), tail length (mm), and mass (g; Appendix 1). We summarized bill and body morphology into a single value using a PPCA in the phytools package for R (Revell 2012). We multiplied body size PPCA values (body PC1) by -1 to help with interpretation of axis loadings (Vehrencamp et al. 2003).
Song characteristics may be under selection to optimize transmission given the vegetation in the environment (Marten and Marler 1977, Marten et al. 1977). To test this hypothesis, we included estimates of forest cover from Mikula et al. (2020) in our models, a similar study that assessed acoustic adaptation of peak song frequency in all Passeriformes birds. Briefly, Mikula et al. (2020) estimated tree cover using data from the Copernicus Global Land Cover project (Buchhorn et al. 2020). For each geographic location of bird song recordings in their study, they extracted the percentage of tree cover in a 100 m² quadrant using the “exactextractr” package (v.0.2.1) in R (Baston 2020). They then calculated species-specific tree cover percentages as the mean of all conspecific recordings in their dataset. In our analyses, we used the mean forest percentage cover for each Tyrannidae species from Mikula et al. (2020). The criteria for song selection in Mikula et al. (2020) was similar to our methodology herein, and the geographic extent broadly overlapped with that in our study, so the forest cover data are well suited for our models to similarly test acoustic adaptation in Tyrannidae. One species was missing forest cover data (Myiarchus validus), so we imputed data using the mean value (62.2%), as implemented in the “mice” package (van Buuren and Groothuis-Oudshoorn 2011) for R (R Core Team 2017). As a further test of acoustic adaptation, we collected breeding ranges (km²) from Tobias et al. (2022), under the assumption that species with larger ranges inhabit more variable habitats (Hawkins and Felizola Diniz-Filho 2006; Appendix 1).
We also included diet breadth in our models because diet may interact with bill and body size to indirectly shape song (Brandl et al. 1994, Brändle et al. 2002, Grant and Grant 2006). We characterized diet breadth for each species by first gathering the percentage of consumption of 6 major food types from Wilman et al. (2014), where the frequency of use for each food type is recorded between 0 (never used) and 100 (approximately used exclusively; Appendix 1). Then we built a similarity matrix of nutritional content for each food type from Sayol et al. (2018) and calculated a breadth index using Rao’s quadratic entropy in the R package “indicspecies” (De Cáceres and Legendre 2009), where larger diet breadth values represent broader diets (Appendix 1). The resource niche values estimate the species’ diets but also account for differences in the resources, making them more accurate diet estimates (De Cáceres et al. 2011). Previous research testing diet differences among species calculated breadth used similar methodology (e.g., Sol et al. 2014).
Phylogenetic methods
To control for phylogenetic non-independence in the analysis, we downloaded 1000 potential phylogenies from https://birdtree.org (Jetz et al. 2012, 2014) for the 282 Tyrannidae species for which we had sufficient data. TreeAnnotator in BEAST v.1.10.1 (Suchard et al. 2018) was used to construct a maximum clade credibility tree using 1% burn in and mean node heights. This approach was used in similar studies (e.g., Pageau et al. 2020).
Statistical analysis
All analyses were performed in R 3.5.3 (R Core Team 2017). To test the relationship between song characteristics and our predictor variables, we built Pagel’s lambda (λ) character evolution models using phylogenetic generalized least square (PGLS) analyses, in the “nlme” package (Pinheiro et al. 2018). This technique uses knowledge of phylogenetic relationships to estimate the expected covariance in cross-species data (Symonds and Blomberg 2014). When using this technique, closely related species are assumed to have similar traits due to their shared ancestry (Symonds and Blomberg 2014), so phylogenetic relationships are controlled within the analysis. Using a stepwise model reduction based on Akaike information criterion (AIC), we examined the factor(s) that best explained song structure. To do so, we first built a full model that included song characteristics (summarized by PPCA) as the response variable, and diet breadth, forest cover, bill and body structure (both summarized by PPCA), range size, and bioclimatic variables as the main effects. The distributions of our main effects were variable, so we scaled all predictors to a mean of 0 prior to analyses. We then performed model selection by testing all possible models using the StepAIC function in the MASS package (Venables and Ripley 2002) and selected the top model based on the change in AIC between the full model and each reduced model (Burnham and Anderson 2002). Changes in AIC values within four of the top models were considered competitive (Burnham and Anderson 2002) and we selected the best fitting model using ANOVAs. We also present Bayesian information criterion (BIC) values and the phylogenetic signal (λ) for the full and final PGLS models. For reduced models, we calculated p-values for main effects using likelihood ratio tests. We then estimated effect sizes (ϕ) and confidence intervals for the final main effects using the “effectsize” package (Ben-Shachar et al. 2020). We also conducted a separate univariate PGLS analysis testing the relationship between song note rate (number of notes divided by song duration and log-transformed) and bill size (summarized by PPCA). We did this because note rate was not captured in our song PPCA but is hypothesized to covary with bill size (Podos and Nowicki 2004, Ballantine 2006). To guard against Type I error, we corrected our α-level for multiple tests of bill size (Bonferroni corrected α = 0.05 divided by 2; corrected α = 0.025).
We then built phylogenetic path models using the R package “phylopath” (van der Bijl 2018) to assess the direct and indirect effects of the variables from the top PGLS models on tyrannid song evolution. We first generated candidate path analysis models informed by the PGLS models and then ranked the path models using an information theory approach based on C-statistics (Shipley 2009). Information theory evaluates the conditional independencies of each model and assigns a C statistic and candidate path models are ranked based on the change in C statistic (ΔCICc) among models. Lower C scores are optimized models, so we selected the top path analysis model with lowest C statistic and competing models within 2 CICc were averaged (van der Bijl 2018).
To assess the relationship between acoustic distance and geographic distance, we constructed pairwise distance matrices between all comparisons of species’ geographic location and their song variables. To calculate acoustic distances, we used the “vegdist” function in the Vegan package for R, using Euclidean distances (Oksanen et al. 2013). We then estimated geographic distances (linear distance in km) between all species comparisons, calculated using the midpoint latitude and longitude value of the recording locations (Schnute et al. 2017, Tobias et al. 2022). Using this method, it is possible centroids may fall outside the breeding range, however we do not think this is likely because songs were recorded during the breeding season. We subsequently conducted a Mantel test, under the hypothesis that Euclidean song distances would be greater if species ranges were closer in proximity (i.e., trait distance is negatively related to geographic distance). Although Mantel tests may suffer from low statistical power (Harmon and Glor 2010), the debate is equivocal (Hardy and Pavoine 2012). It is undisputed however, that Mantel tests in comparative statistical frameworks do not meet assumptions of independence (Harmon and Glor 2010, Hardy and Pavoine 2012), so we addressed this issue by conducting a phylogenetically controlled Mantel test using the R package “evolqg” (Melo et al. 2016). We conducted Mantel tests at two taxonomic scales; first at the family scale and then at the genus-level because congeners are more likely to hybridize. For the genus-level analysis, we only included genera with n ≥ 10 species (restricting us to n = 9 genera) and we treated genera as separate hypotheses.
RESULTS
From our body size PPCA, PC1 explained 75.3% (eigenvalue = 72.1) and PC2 explained 20.96% (eigenvalue = 20.1) of the variation (Appendix 3). Tarsus length, wing length, tail length, and mass all loaded positively on PC1 (Appendix 3), indicating higher body PC1 values represent larger-bodied birds. From our bill size PPCA, PC1 explained 91% (eigenvalue = 83.2) and PC2 explained 6.4% (eigenvalue = 0.06; Appendix 3). Nares bill length, bill width, and bill depth all loaded positively on bill PC1 (Appendix 3). Therefore, higher bill PC1 values represent heftier bills that were longer, wider, and deeper. Body and bill size PC1 explained the majority of the variation and had eigenvalues > 1, so our analyses are based on those PC scores.
From our song PPCA, PC1 explained 55.6% of the variation (eigenvalue = 6.76) and PC2 explained 26.8% (eigenvalue = 6.25; Appendix 4). Song PC1 was positively correlated with frequency characteristics (mean frequency, mean peak frequency, and maximum dominant frequency), indicating lower song PC1 values represented lower frequency songs. Song PC2 was positively correlated with temporal characteristics of tyrannid songs including song duration and number of notes (Appendix 4). Therefore, lower song PC2 values represented shorter songs with fewer notes. Cumulatively, song PC1 and PC2 explained most of the variation and had eigenvalues > 1, so we used these PC scores in subsequent analyses.
The final model predicting song PC1 (Table 1, representing song frequency characteristics) included body PC1 (X²1279 = 11.10, p = 0.0008, ϕ = 0.19 [CI: 0.06-0.31]) and diet breadth (X21279 = 5.39, p = 0.02, ϕ = 0.12 [CI: 0-0.25]), however effect size CIs included 0 for diet breadth (Table 2). As predicted, song PC1 was negatively correlated with body PC1 and negatively correlated with diet breadth (Fig. 2). The final model predicting song PC2 (Table 1, representing song temporal characteristics) included bill PC1 (X21279 = 16.88, p = 0.13, ϕ = 0.07 [CI: 0-0.20]) and mean annual temperature (X21279 = 0.03, p = 0.12, ϕ = 0.07 [CI: 0-0.20]), however both predictors were not significant (p > 0.05) and effect size CIs included 0 (Table 2). From a separate univariate Bonferroni corrected PGLS analysis, larger-billed species produced slower paced songs, as note rate was negatively related to bill PC1 (Fig. 3; X21279 = 13.36, p = 0.0003; ϕ = 0.21 [CI: 0.08-0.33]; Table 2).
Our path analyses revealed consistent effects of morphology, ecology, and climate on song frequency and temporal elements (Appendix 5). For song PC1, we identified body size and diet breadth as having overall negative effects (coeff. = -0.28 and -0.10, respectively), and larger-bodied birds had broader diets (Fig. 4). Bill size had a direct positive effect on song PC2 (coeff. = 0.12) and birds with larger bills were found in colder environments, thus having an indirect negative influence on song PC2 (coeff. = -0.04; Fig. 4).
We found mixed support for the species recognition hypothesis (Table 3). First, we conducted a phylogenetic Mantel test that included all Tyrannidae in our study and found no relationship between pairwise song and geographic distances (Appendix 6; r = -0.08, p = 0.99, [CI: -0.09 - -0.07]). However, the CIs from this test did not overlap zero, suggesting a negative relationship albeit weak (Table 3). We then conducted phylogenetic Mantel tests within the genus-level and found Tyrannus song was positively related to geographic distance (Table 3; r = 0.53, p = 0.02, [CI: 0.33-0.68]). We found a non-significant relationship in Myiarchus and Poecilotriccus song and proximity (Table 3; Myiarchus: r = -0.19, p = 0.87, [CI: -0.37 - 0.0]; Poecilotriccus: r = 0.38, p = 0.10, [CI: 0.10 - 0.61]), however CIs for Poecilotriccus did not overlap zero suggesting a positive relationship. All other genera showed no statistical relationship between pairwise song and geographic distances (Table 3).
DISCUSSION
Vocalizations are an important communication modality across animal taxa, and in birds, song is used for mate attraction, territory defense, and other key social interactions (Collins 2004). Because of its critical function in bird biology and ecology, there is much interest in understanding the evolutionary influences of song. In our study, we simultaneously tested morphological, ecological, and geographic explanations of song variation in Tyrannidae, a speciose suboscine passeriform family, using a large-scale dataset of the Americas. From PGLS and phylogenetic path analyses, we found strong support for the morphological adaptation hypothesis; Tyrannid song frequency was largely driven by morphology, and our phylogenetic path analysis revealed body size had a relatively strong effect, where larger-bodied species produced lower frequency songs (Mason and Burns 2015, Derryberry et al. 2018, Mikula et al. 2020, Demery et al. 2021). Bird body size covaries with aspects of the vocal apparatus, such as the size of the vibratory structures, the vocal tract, and the size of the labia in the syrinx (Podos 2001, Riede and Goller 2010, Mikula et al. 2020), so larger syrinxes in tyrannids may result in lower frequency songs.
Bill morphology may also influence song, where birds with relatively larger and stronger bills will face performance constraints on their vocal tract (Podos 2001). As jaws become more adapted for strength, for example to crush harder foods, they will be less able to perform the rapid movements required for high pitched trills (Podos 2001). Based on our PGLS analysis, tyrannid bill morphology was not related to song PC2 (representing temporal elements), as expected due to mechanical limitations of larger bills (Podos 2001). Our path analysis revealed a direct positive effect of bill size on temporal characteristics, consistent with previous work showing larger bills produce longer notes and songs (Demery et al. 2021). The effect was weak however (path coefficient = 0.12), perhaps because flycatcher bill morphology does not vary as widely as body size (e.g., bill width SD = 2.04 mm, bill depth SD = 1.6 mm; wing length SD = 22.2 mm, body mass SD = 12.3 g). We separately tested the relationship between bill size and note rate, a vocal characteristic sensitive to bill size variation (Podos 2001) and found a negative effect as in previous studies (Podos and Nowicki 2004, Ballantine 2006, Derryberry 2009). Taken together, our results are therefore consistent with the notion that ecological selection for body and bill morphology may shape song in Tyrannidae (Grant and Grant 2006).
Climate temperature was identified in the final PGLS model for Tyrannidae song temporal elements, however the effect was weak and indirect. Along with greater precipitation, warmer temperature may increase vegetation growth, creating selection for vocalizations that optimize transmission via sensory drive (Boncoraglio and Saino 2007, Medina and Francis 2012). A systematic review of sensory drive studies over 25 years found that in 132 studies, 86% supported the hypothesis (Cummings and Endler 2018). Our study included estimates of forest cover to test this hypothesis, however we found no evidence of sensory drive. Internote interval may be a more sensitive temporal element to degradation in dense vegetation, but we did not quantify this because some species in our dataset produced single note songs. Sensory drive is confounded in part because signals are produced by senders and heard by receivers, and selection can work on both the signaling and auditory components, in different ways (Mason and Burns 2015). Of the studies focused on auditory signals in birds, 21 (80.7%) found support for the signaling aspect of the hypothesis and 1 found support for the sensory components in the sensory drive hypothesis. Among the studies supporting auditory sensory drive, there was mixed evidence for the signaling component in Ash-throated Flycatchers (M. cinerascens) whereas Gray Flycatcher (Empidonax wrightii) song showed no relationship with environmental effects on song (Francis et al. 2010).
We assessed the species recognition hypothesis at two taxonomic scales and found mixed support. Overall, pairwise song differences showed weakly negative effects with heterospecific proximity. This result is consistent with findings from previous work on species recognition, in which species located in closer proximity face pressure to differentiate themselves, either to prevent hybridization or to minimize heterospecific signal overlap (Liou and Price 1994, Seddon 2005, Simpson et al. 2021). We also assessed the relationship between song differences and geographic distance within genera because congeners are more likely to hybridize. Tyrannus, Myiarchus, and Poecilotriccus were the only genera to show pronounced pairwise song differences, perhaps to prevent costly hybridizations because their plumage color does not vary widely among congeners (Rheindt et al. 2008), for example, Western (T. verticalis) vs. Cassin’s Kingbird (T. vociferans) and Brown-crested (M. tyrannulus) vs. Ash-throated Flycatcher. Alternatively, because Tyrannus and Poecilotriccus song differences were positively related to distance, this may instead indicate genetic drift effects (Irwin et al. 2001). Interestingly, song differences in other groups with conserved coloration did not show effects of range proximity. One genus with notoriously conserved plumage is Empidonax (Johnson and Cicero 2002, but see Part II of our study), where some heterospecifics are so morphologically similar they were previously thought to be conspecifics (Prescott 1987). One should therefore expect to find Empidonax song to predictably covary with distance among congeners. We however found no relationship between song differences and range proximity in Empidonax. In fact, the majority of genera from our study did not show a relationship between song differences and geographic distance. Perhaps flycatcher taxa use other communication modalities, for example body size, plumage structure, and plumage ornaments such as exaggerated crowns or tail feathers to discern conspecifics (West-Eberhard 1983). Alternatively, behavioral differences may help discern heterospecifics (Luther 2009, Maynard et al. 2012). Future studies assessing the degree of range overlap (Simpson et al. 2021) and morphological divergence (Kirschel et al. 2009) would be helpful to unravel the modality driving tyrannid species recognition. Despite the weak negative correlation of song and distance, the effect was more variable in plumage color (see Part II of our study), so overall our results are consistent with the notion of species recognition via vocalizations as a driving force in Tyrannidae evolution.
In conclusion, we found song evolution in the largest Passeriformes family, Tyrannidae, was largely driven by morphology in which larger-bodied species with heftier bills sang lower frequency and slower paced songs. Song frequency was also affected by diet breadth, perhaps because species with broader diets had larger bodies and bills. This is consistent with the literature on song divergence and further supports the hypothesis that larger syrinxes produce lower frequency songs (Podos and Nowicki 2004, Ballantine 2006, Riede and Goller 2010). Bill morphology was not related to other song temporal traits, as expected due to physiological limitations of larger bills (Podos 2001), perhaps because bill size variation is more consistent among heterospecifics relative to body size. There was also no support for sensory drive because vegetation and climate variables did not strongly influence song characteristics. Finally, we found support for the species recognition hypothesis, but song frequency and temporal characteristics were weakly negatively correlated with the proximity of heterospecifics and the effect varied among the genera we tested. Overall, the results from our study indicate the evolution of song in Tyrannidae is primarily constrained by morphology and ecology, possibly influenced by genetic drift, or used to reinforce species boundaries in a family in which many members exhibit conserved plumage coloration.
RESPONSES TO THIS ARTICLE
Responses to this article are invited. If accepted for publication, your response will be hyperlinked to the article. To submit a response, follow this link. To read responses already accepted, follow this link.
AUTHOR CONTRIBUTIONS
Equal contributions to lead authorship: JS and SMM. SMM conceived of the project. JS and SM collected and quantified song recordings. SMM conducted song analyses. JS and SMM conducted the statistical analysis. QJ assisted with analyses. JS wrote initial draft and SMM wrote final draft. MWR assisted in writing and analysis. All authors contributed to editing the manuscript and gave final approval for the manuscript.
ACKNOWLEDGMENTS
This research was completed on the traditional lands of the Tk’emlúps te Secwépemc within Secwépemc’ulucw, the traditional and unceded territory of the Secwépemc. Funding for this project was provided by a Natural Sciences and Engineering Research Council of Canada Discovery Grant to M. W. R. We thank xeno-canto and Cornell Lab of Ornithology for access to their song recording libraries and to iNaturalist users for their photos in Fig. 1 (zacsf: Elaenia gigas Photo 52736945; shrike2: Attila cinnamomeus Photo 30969805). Suggestions from M. Hauber, two anonymous reviewers, and M. Shaikh greatly improved the manuscript.
DATA AVAILABILITY
Data used in this study are available on Figshare.
LITERATURE CITED
Araya-Salas, M., and G. Smith-Vidaurre. 2017. warbleR: an R package to streamline analysis of animal acoustic signals. Methods in Ecology and Evolution 8:184-191. https://dx.doi.org/10.1111/2041-210X.12624
Baston, D. 2020. exactextractr: fast extraction from raster datasets using polygons. R package version 0.5.0. R Foundation for Statistical Computing, Vienna, Austria. https://cran.r-project.org/web/packages/exactextractr/index.html
Ballentine, B. 2006. Morphological adaptation influences the evolution of a mating signal. Evolution 60:1936-1944. https://doi.org/10.1111/j.0014-3820.2006.tb00536.x
Ben-Shachar, M. S., D. Lüdecke, and D. Makowski. 2020. effectsize: estimation of effect size indices and standardized parameters. Journal of Open Source Software 5:2815. https://doi.org/10.21105/joss.02815
Boncoraglio, G., and N. Saino. 2007. Habitat structure and the evolution of bird song: a meta-analysis of the evidence for the acoustic adaptation hypothesis. Functional Ecology 21:134-142. https://doi.org/10.1111/j.1365-2435.2006.01207.x
Bradbury, J. W., and S. L. Vehrencamp. 1998. Principles of animal communication. Second edition. Sinauer Associates, Sunderland, Massachusetts, USA.
Brandl, R., A. Kristín, and B. Leisler. 1994. Dietary niche breadth in a local community of passerine birds: an analysis using phylogenetic contrasts. Oecologia 1:109-116. https://doi.org/10.1007/BF00326096
Brändle, M., A. Prinzing, R. Pfeifer, and R. Brandl. 2002. Dietary niche breadth for Central European birds: correlations with species-specific traits. Evolutionary Ecology Research 4:643-657. https://www.evolutionary-ecology.com/issues/v04n05/ddar1364.pdf
Buchhorn, M., M. Lesiv, N.-E. Tsendbazar, M. Herold, L. Bertels, and B. Smets. 2020. Copernicus global land cover layers — collection 2. Remote Sensing 12:1044. https://doi.org/10.3390/rs12061044
Burnham, K. P., and D. R. Anderson. 2002. Model selection and multi-model inference: a practical information-theoretic approach. Second edition. Springer, New York, New York, USA. https://doi.org/10.1007/b97636
Cicero, C., and N. K. Johnson. 2002. Phylogeny and character evolution in the Empidonax group of tyrant flycatchers (Aves: Tyrannidae): a test of W. E. Lanyon’s hypothesis using mtDNA sequences. Molecular Phylogenetics and Evolution 22:289-302. https://doi.org/10.1006/mpev.2001.1054
Clayton, D. F., C. N. Balakrishnan, and S. E. London. 2009. Integrating genomes, brain and behavior in the study of songbirds. Current Biology 19:R865-R873. https://doi.org/10.1016/j.cub.2009.07.006
Collins, S. 2004. Vocal fighting and flirting: the functions of birdsong. Pages 39-79 in P. Marler and H. Slabbekoorn, editors. Nature’s music: the science of birdsong. Elsevier Academic, San Diego, California, USA. https://doi.org/10.1016/B978-012473070-0/50005-0
Cummings, M. E., and J. A. Endler. 2018. 25 years of sensory drive: the evidence and its watery bias. Current Zoology 64:471-484. https://doi.org/10.1093/cz/zoy043
De Céceres, M., and P. Legendre. 2009. Associations between species and groups of sites: indices and statistical inference. Ecology 90:3566-3574. https://doi.org/10.1890/08-1823.1
De Céceres, M., D. Sol, O. Lapiedra, and P. Legendre. 2011. A framework for estimating niche metrics using the resemblance between qualitative resources. Oikos 120:1341-1350. https://doi.org/10.1111/j.1600-0706.2011.19679.x
Demery, A.-J. C., K. J. Burns, and N. A. Mason. 2021. Bill size, bill shape, and body size constrain bird song evolution on a macroevolutionary scale. Auk 138:ukab011. https://doi.org/10.1093/ornithology/ukab011
Derryberry, E. P. 2009. Ecology shapes birdsong evolution: variation in morphology and habitat explains variation in White-crowned Sparrow song. American Naturalist 174:24-33. https://doi.org/10.1086/599298
Derryberry, E. P., N. Seddon, G. E. Derryberry, S. Claramunt, G. F. Seeholzer, R. T. Brumfield, and J. A. Tobias. 2018. Ecological drivers of song evolution in birds: disentangling the effects of habitat and morphology. Ecology and Evolution 8:1890-1905. https://doi.org/10.1002/ece3.3760
Dynesius, M., and R. Jansson. 2000. Evolutionary consequences of changes in species’ geographical distributions driven by Milankovitch climate oscillations. Proceedings of the National Academy of Sciences 97:9115-9120. https://doi.org/10.1073/pnas.97.16.9115
Endler, J. A. 1980. Natural selection on color patterns in Poecilia reticulata. Evolution 34:76-91. https://doi.org/10.1111/j.1558-5646.1980.tb04790.x
Endler, J. A. 1992. Signals, signal conditions, and the direction of evolution. American Naturalist 139:S125-S153. https://doi.org/10.1086/285308
Fick, S. E., and R. J. Hijmans. 2017. WorldClim 2: new 1-km spatial resolution climate surfaces for global land areas. International Journal of Climatology 37:4302-4315. https://doi.org/10.1002/joc.5086
Francis, C. D., C. P. Ortega, and A. Cruz. 2010. Vocal frequency change reflects different responses to anthropogenic noise in two suboscine tyrant flycatchers. Proceedings of the Royal Society of London: B 278:2025-2031. https://doi.org/10.1098/rspb.2010.1847
Francis, C. D., and M. R. Wilkins. 2021. Testing the strength and direction of selection on vocal frequency using metabolic scaling theory. Ecosphere 12:e03733. https://doi.org/10.1002/ecs2.3733
Freeman, B. G., G. A. Montgomery, and D. Schluter. 2017. Evolution and plasticity: divergence of song discrimination is faster in birds with innate song than in song learners in Neotropical passerine birds. Evolution 71:2230-2242. https://doi.org/10.1111/evo.13311
Grant, P. R., and B. R. Grant. 2006. Evolution of character displacement in Darwin’s finches. Science 313:224-226. https://doi.org/10.1126/science.1128374
Hardy, O. J., and S. Pavoine. 2012. Assessing phylogenetic signal with measurement error: a comparison of mantel tests, Blomberg et al.’s k, and phylogenetic distograms. Evolution 66:2614-2621. https://doi.org/10.1111/j.1558-5646.2012.01623.x
Harmon, L. J., and R. E. Glor. 2010. Poor statistical performance of the Mantel test in phylogenetic comparative analyses. Evolution 64:2173-2178. https://doi.org/10.1111/j.1558-5646.2010.00973.x
Hawkins, B. A., and J. A. Felizola Diniz‐Filho. 2006. Beyond Rapoport’s rule: evaluating range size patterns of New World birds in a two‐dimensional framework. Global Ecology and Biogeography 15:461-469. https://doi.org/10.1111/j.1466-822X.2006.00243.x
Irwin, D. E., S. Bensch, and T. D. Price. 2001. Speciation in a ring. Nature 409:333-337. https://doi.org/10.1038/35053059
Jetz, W., G. H. Thomas, J. B. Joy, K. Hartmann, and A. O. Mooers. 2012. The global diversity of birds in space and time. Nature 491:444-448. https://doi.org/10.1038/nature11631
Jetz, W., G. H. Thomas, J. B. Joy, D. W. Redding, K. Hartmann, and A. O. Mooers. 2014. Global distribution and conservation of evolutionary distinctness in birds. Current Biology 24:919-930. https://doi.org/10.1016/j.cub.2014.03.011
Johnson, N. K., and C. Cicero. 2002. The role of ecologic diversification in sibling speciation of Empidonax flycatchers (Tyrannidae): multigene evidence from mtDNA. Molecular Ecology 11:2065-2081. https://doi.org/10.1046/j.1365-294X.2002.01588.x
Kirschel, A. N., D. T. Blumstein, and T. B. Smith. 2009. Character displacement of song and morphology in African tinkerbirds. Proceedings of the National Academy of Sciences 106:8256-8261. https://doi.org/10.1073/pnas.0810124106
Liou, L. W., and T. D. Price. 1994. Speciation by reinforcement of premating isolation. Evolution 48:1451-1459. https://doi.org/10.1111/j.1558-5646.1994.tb02187.x
Liu, W.-C., K. Wada, E. D. Jarvis, and F. Nottebohm. 2013. Rudimentary substrates for vocal learning in a suboscine. Nature Communications 4:2082. https://doi.org/10.1038/ncomms3082
Luther, D. 2009. The influence of the acoustic community on songs of birds in a neotropical rain forest. Behavioral Ecology 20:864-871. https://doi.org/10.1093/beheco/arp074
Marten, K., and P. Marler. 1977. Sound transmission and its significance for animal vocalization. Behavioral Ecology and Sociobiology 2:271-290. https://doi.org/10.1007/BF00299740
Marten, K., D. Quine, and P. Marler. 1977. Sound transmission and its significance for animal vocalization: II. Tropical forest habitats. Behavioral Ecology and Sociobiology 2:291-302. https://doi.org/10.1007/BF00299741
Mason, N. A., and K. J. Burns. 2015. The effect of habitat and body size on the evolution of vocal displays in Thraupidae (tanagers), the largest family of songbirds. Biological Journal of the Linnaean Society 114:538-551. https://doi.org/10.1111/bij.12455
Maynard, D. F., K.-A. A. Ward, S. M. Doucet, and D. J. Mennill. 2012. Calling in an acoustically competitive environment: duetting male long-tailed manakins avoid overlapping neighbours but not playback-simulated rivals. Animal Behaviour 84:563-573. https://doi.org/10.1016/j.anbehav.2012.06.008
Mayr, E. 1942. Systematics and the origin of species. Columbia University Press, New York, New York, USA.
Medina, I., and C. D. Francis. 2012. Environmental variability and acoustic signals: a multi-level approach in songbirds. Biology Letters 8:928-931. https://doi.org/10.1098/rsbl.2012.0522
Melo, D., G. Garcia, A. Hubbe, A. P. Assis, and G. Marroig. 2016. EvolQG - an R package for evolutionary quantitative genetics. F1000Research 4:925. https://doi.org/10.12688/f1000research.7082.2
Mikula, P., M. Valcu, H. Brumm, M. Bulla, W. Forstmeier, T. Petrusková, B. Kempenaers, and T. Albrecht. 2020. A global analysis of song frequency in passerines provides no support for the acoustic adaptation hypothesis but suggests a role for sexual selection. Ecology Letters 24:477-486. https://doi.org/10.1111/ele.13662
Morton, E. S. 1975. Ecological sources of selection on avian sounds. American Naturalist 109:17-34. https://doi.org/10.1086/282971
Nicholls, J. A., and A. W. Goldizen. 2006. Habitat type and density influence vocal signal design in Satin Bowerbirds. Journal of Animal Ecology 75:549-558. https://doi.org/10.1111/j.1365-2656.2006.01075.x
Nuñez, M. A., M. C. Chiuffo, A. Pauchard, and R. D. Zenni. 2021. Making ecology really global. Trends in Ecology and Evolution 36:766-769. https://doi.org/10.1016/j.tree.2021.06.004
Odom, K. J., and L. Benedict. 2018. A call to document female bird songs: applications for diverse fields. Auk 35:314-325. https://doi.org/10.1642/AUK-17-183.1
Oksanen, J., F. G. Blanchet, R. Kindt, P. Legendre, P. R. Minchin, R. B. O’hara, G. L. Simpson, P. Solymos, M. H. H. Stevens, and H. Wagner. 2013. Package ‘vegan’ community ecology package, Version 2. R Foundation for Statistical Computing, Vienna, Austria. https://cran.r-project.org/web/packages/vegan/vegan.pdf
Pageau, C., C. M. Tonra, M. Shaikh, N. J. Flood, and M. W. Reudink. 2020. Evolution of moult-migration is directly linked to aridity of the breeding grounds in North American passerines. Biology Letters 16:20200155. https://doi.org/10.1098/rsbl.2020.0155
Pigot, A. L., C. Sheard, E. T. Miller, T. P. Bregman, B. G. Freeman, U. Roll, N. Seddon, C. H. Trisos, B. C. Weeks, and J. A. Tobias. 2020. Macroevolutionary convergence connects morphological form to ecological function in birds. Nature Ecology and Evolution 4:230-239. https://doi.org/10.1038/s41559-019-1070-4
Pinheiro, J., D. Bates, S. DebRoy, and D. Sarkar. 2018. nlme: linear and nonlinear mixed effects models. R package version 3. R Foundation for Statistical Computing, Vienna, Austria. https://cran.r-project.org/web/packages/nlme/nlme.pdf
Podos, J. 2001. Correlated evolution of morphology and vocal signal structure in Darwin’s finches. Nature 409:185-188. https://doi.org/10.1038/35051570
Podos, J., and S. Nowicki. 2004. Beaks, adaptation, and vocal evolution in Darwin’s finches. Bioscience 54:501-510. https://doi.org/10.1641/0006-3568(2004)054[0501:BAAVEI]2.0.CO;2
Prescott, D. R. C. 1987. Territorial responses to song playback in allopatric and sympatric populations of Alder Empidonax alnorum and Willow E. traillii flycatchers. Wilson Bulletin 99:611-619.
Prum, R. O. 2010. The Lande-Kirkpatrick mechanism is the null model of evolution by intersexual selection: implications for meaning, honesty, and design in intersexual signals. Evolution: International Journal of Organic Evolution 64:3085-3100. https://doi.org/10.1111/j.1558-5646.2010.01054.x
R Core Team. 2017. R: a language and environment for statistical computing. R Foundation for Statistical Computing, Vienna, Austria. https://www.R-project.org
Revell, L. J. 2012. phytools: an R package for phylogenetic comparative biology (and other things). Methods in Ecology and Evolution 3:217-223. https://doi.org/10.1111/j.2041-210X.2011.00169.x
Rheindt, F. E., J. A. Norman, and L. Christidis. 2008. DNA evidence shows vocalizations to be a better indicator of taxonomic limits than plumage patterns in Zimmerius tyrant-flycatchers. Molecular Phylogenetics and Evolution 48:150-156. https://doi.org/10.1016/j.ympev.2008.04.016
Richards, D. G., and R. H. Wiley. 1980. Reverberations and amplitude fluctuations in the propagation of sound in a forest: implications for animal communication. American Naturalist 115:381-399. https://doi.org/10.1086/283568
Riebel, K., K. J. Odom, N. E. Langmore, and M. L. Hall. 2019. New insights from female bird song: towards an integrated approach to studying male and female communication roles. Biology Letters 15:20190059. https://doi.org/10.1098/rsbl.2019.0059
Riede, T., and F. Goller. 2010. Functional morphology of the sound-generating labia in the syrinx of two songbird species. Journal of Anatomy 216:23-36. https://doi.org/10.1111/j.1469-7580.2009.01161.x
Sayol, F., P. A. Downing, A. N. Iwaniuk, J. Maspons, and D. Sol. 2018. Predictable evolution towards larger brains in birds colonizing oceanic islands. Nature Communications 9:2820. https://doi.org/10.1038/s41467-018-05280-8
Schnute, J. T., N. M. Boers, R. Haigh, and A. Couture-Beil. 2017. PBSmapping 2.70.3: user’s guide. Revised from Canadian technical report of fisheries and aquatic sciences 2549. Fisheries and Oceans, Canada, Ottawa, Ontario, Canada.
Sebastianelli, M., S. M. Lukhele, E. C. Nwankwo, L. Hadjioannou, and A. N. G. Kirschel. 2022. Continent‐wide patterns of song variation predicted by classical rules of biogeography. Ecology Letters 25:2448-2462. https://doi.org/10.1111/ele.14102
Seddon, N. 2005. Ecological Adaptation and species recognition drives vocal evolution in neotropical suboscine birds. Evolution 59:200-215. https://doi.org/10.1111/j.0014-3820.2005.tb00906.x
Shipley, B. 2009. Confirmatory path analysis in a generalized multilevel context. Ecology 90:363-368. https://doi.org/10.1890/08-1034.1
Simpson, R. K., D. R. Wilson, A. F. Mistakidis, D. J. Mennill, and S. M Doucet. 2021. Sympatry drives colour and song evolution in wood-warblers (Parulidae). Proceedings of the Royal Society of London: B 288:20202804. https://doi.org/10.1098/rspb.2020.2804
Sol, D., C. González‐Lagos, D. Moreira, J. Maspons, and O. Lapiedra. 2014. Urbanisation tolerance and the loss of avian diversity. Ecology Letters 17:942-950. https://doi.org/10.1111/ele.12297
Suchard, M. A., P. Lemey, G. Baele, D. L. Ayres, A. J. Drummond, and A. Rambaut. 2018. Bayesian phylogenetic and phylodynamic data integration using BEAST 1.10. Virus Evolution 4::vey016. https://doi.org/10.1093/ve/vey016
Symonds, M. R. E., and S. P. Blomberg. 2014. A primer on phylogenetic generalised least squares. Pages 105-130 in L. Garamszegi, editor. Modern phylogenetic comparative methods and their application in evolutionary biology. Springer, Berlin, Germany. https://doi.org/10.1007/978-3-662-43550-2_5
Tobias, J. A., J. D. Brawn, R. T. Brumfield, E. P. Derryberry, A. N. G. Kirschel, and N. Seddon. 2012. The importance of neotropical suboscine birds as study systems in ecology and evolution. Ornitologia Neotropical 23:259-272.
Tobias, J. A., C. Sheard, A. L. Pigot, A. J. Devenish, J. Yang, F. Sayol, M. H. C. Neate-Clegg, N. Alioravainen, T. L. Weeks, R. A. Barber, P. A. Walkden, H. E. A. MacGregor, S. E. I. Jones, C. Vincent, A. G. Phillips, N. M. Marples, F. A. Montaño-Centellas, V. Leandro-Silva, S. Claramunt, B. Darski, B. G. Freeman, T. P. Bregman, C. R. Cooney, E. C. Hughes, E. J. R. Capp, Z. K. Varley, N. R. Friedman, H. Korntheuer, A. Corrales-Vargas, C. H. Trisos, B. C. Weeks, D. M. Hanz, T. Töpfer, G. A. Bravo, V. Remeš, L. Nowak, L. S. Carneiro, A. J. Moncada R., B. Matysioková, D. T. Baldassarre, A. Martínez-Salinas, J. D. Wolfe, P. M. Chapman, B. G. Daly, M. C. Sorensen, A. Neu, M. A. Ford, R. J. Mayhew, L. F. Silveira, D. J. Kelly, N. N. D. Annorbah, H. S. Pollock, A. M. Grabowska-Zhang, J. P. McEntee, J. C. T. Gonzalez, C. G. Meneses, M. C. Muñoz, L. L. Powell, G. A. Jamie, T. J. Matthews, O. Johnson, G. R. R. Brito, K. Zyskowski, R. Crates, M. G. Harvey, M. J. Zevallos, P. A. Hosner, T. Bradfer-Lawrence, J. M. Maley, F. G. Stiles, H. S. Lima, K. L. Provost, M. Chibesa, M. Mashao, J. T. Howard, E. Mlamba, M. A. H. Chua, B. Li, M. I. Gómez, N. C. García, M. Päckert, J. Fuchs, J. R. Ali, E. P. Derryberry, M. L. Carlson, R. C. Urriza, K. E. Brzeski, D. M. Prawiradilaga, M. J. Rayner, E. T. Miller, R. C. K. Bowie, R.-M. Lafontaine, R. P. Scofield, Y. Lou, L. Somarathna, D. Lepage, M. Illif, E. L. Neuschulz, M. Templin, D. M. Dehling, J. C. Cooper, O. S. G. Pauwels, K. Analuddin, J. Fjeldså, N. Seddon, P. R. Sweet, F. A. J. DeClerck, L. N. Naka, J. D. Brawn, A. Aleixo, K. Böhning-Gaese, C. Rahbek, S. A. Fritz, G. H. Thomas, and M. Schleuning. 2022. AVONET: morphological, ecological and geographical data for all birds. Ecology Letters 25:581- 597. https://doi.org/10.1111/ele.13898
van Buuren, S., and K. Groothuis-Oudshoorn. 2011. mice: multivariate imputation by chained equations in R. Journal of Statistical Software 45:1-67. https://doi.org/10.18637/jss.v045.i03
van der Bijl, W. 2018. phylopath: easy phylogenetic path analysis in R. PeerJ 6:e4718. https://doi.org/10.7717/peerj.4718
Vehrencamp, S. L., A. F. Ritter, M. Keever, and J. W. Bradbury. 2003. Responses to playback of local vs. distant contact calls in the Orange-fronted Conure, Aratinga canicularis. Ethology 109:37-54. https://doi.org/10.1046/j.1439-0310.2003.00850.x
Venables, W. N., and B. D. Ripley. 2002. Modern applied statistics with S. Fourth edition. Springer, New York, New York, USA. https://doi.org/10.1007/978-0-387-21706-2
West-Eberhard, M. J. 1983. Sexual selection, social competition, and speciation. Quarterly Review of Biology 58:155-183. https://doi.org/10.1086/413215
Wiley, R. H. 1991. Associations of song properties with habitats for territorial oscine birds of eastern North America. American Naturalist 138:973-993. https://doi.org/10.1086/285263
Wiley, R. H., and D. G. Richards. 1978. Physical constraints on acoustic communication in the atmosphere: implications for the evolution of animal vocalizations. Behavioral Ecology and Sociobiology 3:69-94. https://doi.org/10.1007/BF00300047
Wilkins, M. R., N. Seddon, and R. J. Safran. 2013. Evolutionary divergence in acoustic signals: causes and consequences. Trends in Ecology and Evolution 28:156-166. https://doi.org/10.1016/j.tree.2012.10.002
Wilman, H., J. Belmaker, J. Simpson, C. de la Rosa, M. M. Rivadeneira, and W. Jetz. 2014. EltonTraits 1.0: species‐level foraging attributes of the world’s birds and mammals: Ecological Archives E095‐178. Ecology 95:2027. https://doi.org/10.1890/13-1917.1
Winkler, D. W., S. M. Billerman, and I. J. Lovette. 2020. Tyrant Flycatchers (Tyrannidae), version 1.0. In S. M. Billerman, B. K. Keeney, P. G. Rodewald, and T. S. Schulenberg, editors. Birds of the world. Cornell Lab of Ornithology, Ithaca, New York, USA. https://doi.org/10.2173/bow.tyrann2.01
Fig. 1
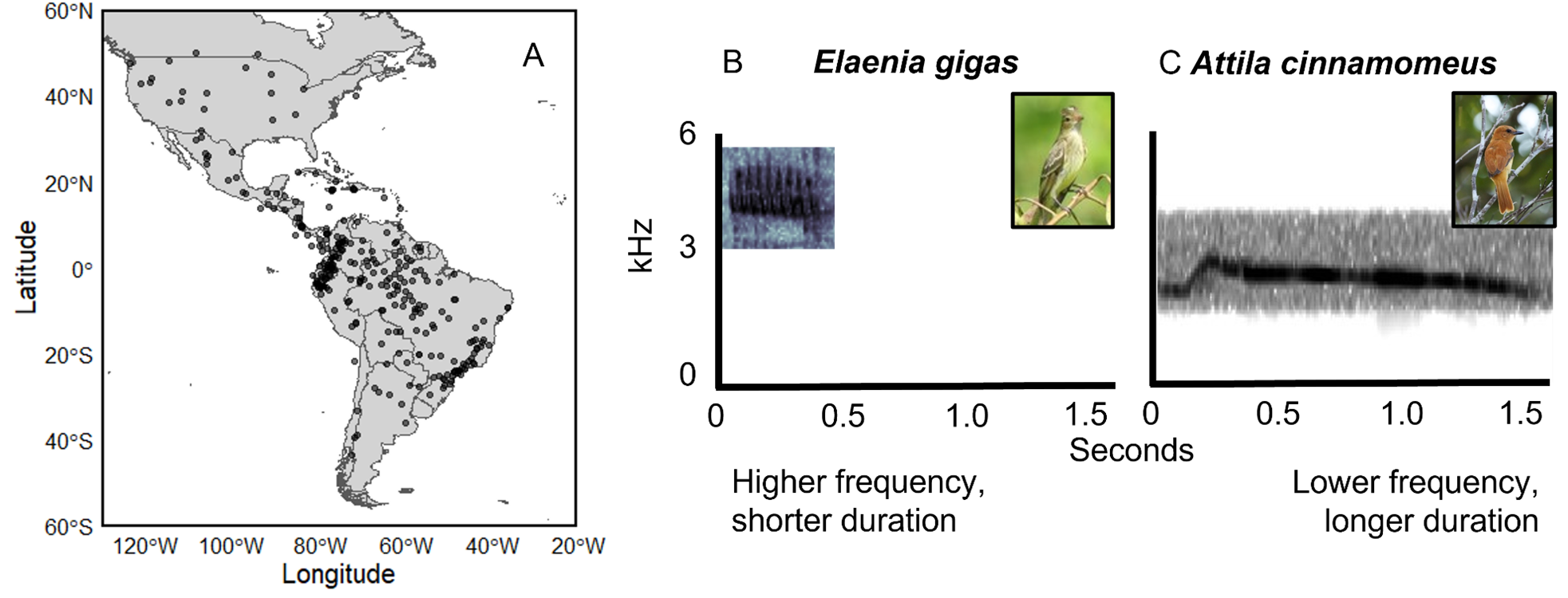
Fig. 1. A. Suboscine Tyrannidae species occur across broad latitudinal, climatic, and ecological gradients (recording location centroids for each species [n = 282] are shown by black dots). Song among heterospecifics is variable in frequency and temporal aspects (B-C), but the factors driving song evolution is unresolved. Photo credits: B. Elaenia gigas (iNaturalist user zacsf), C. Attila cinnamomeus (iNaturalist user shrike2). Links to photos are in the acknowledgments.
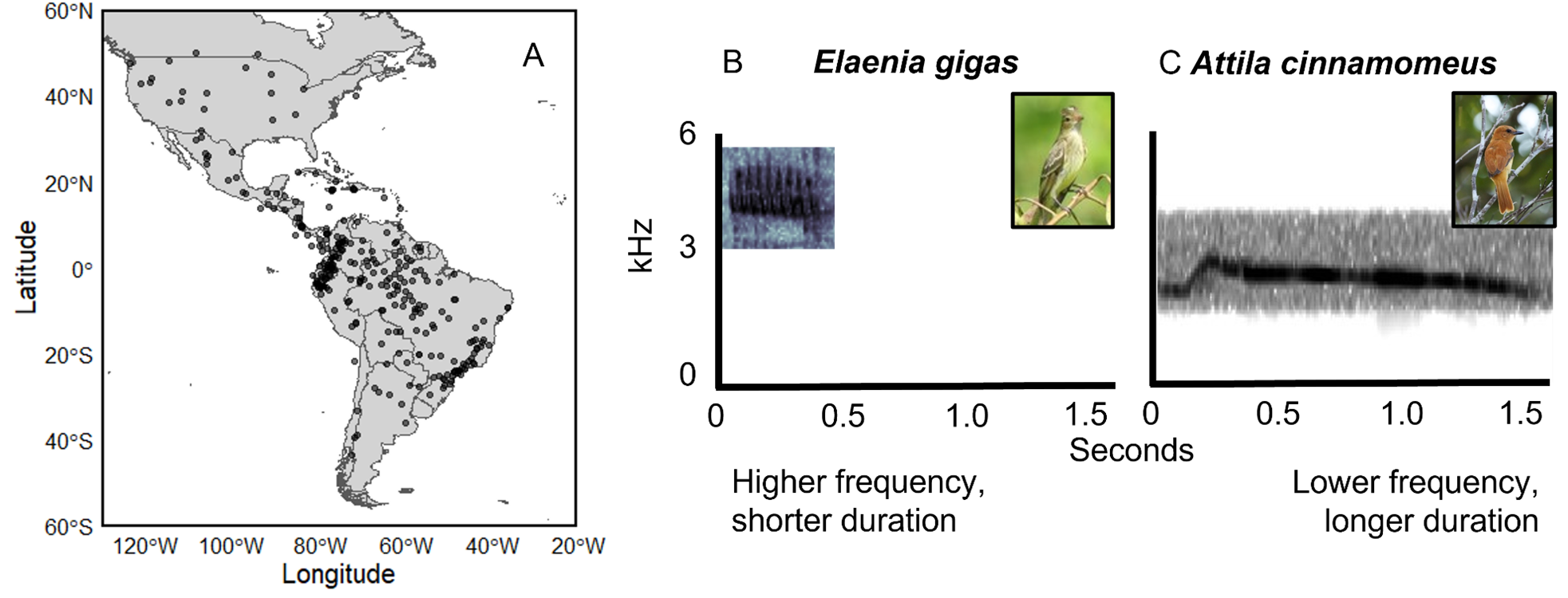
Fig. 2
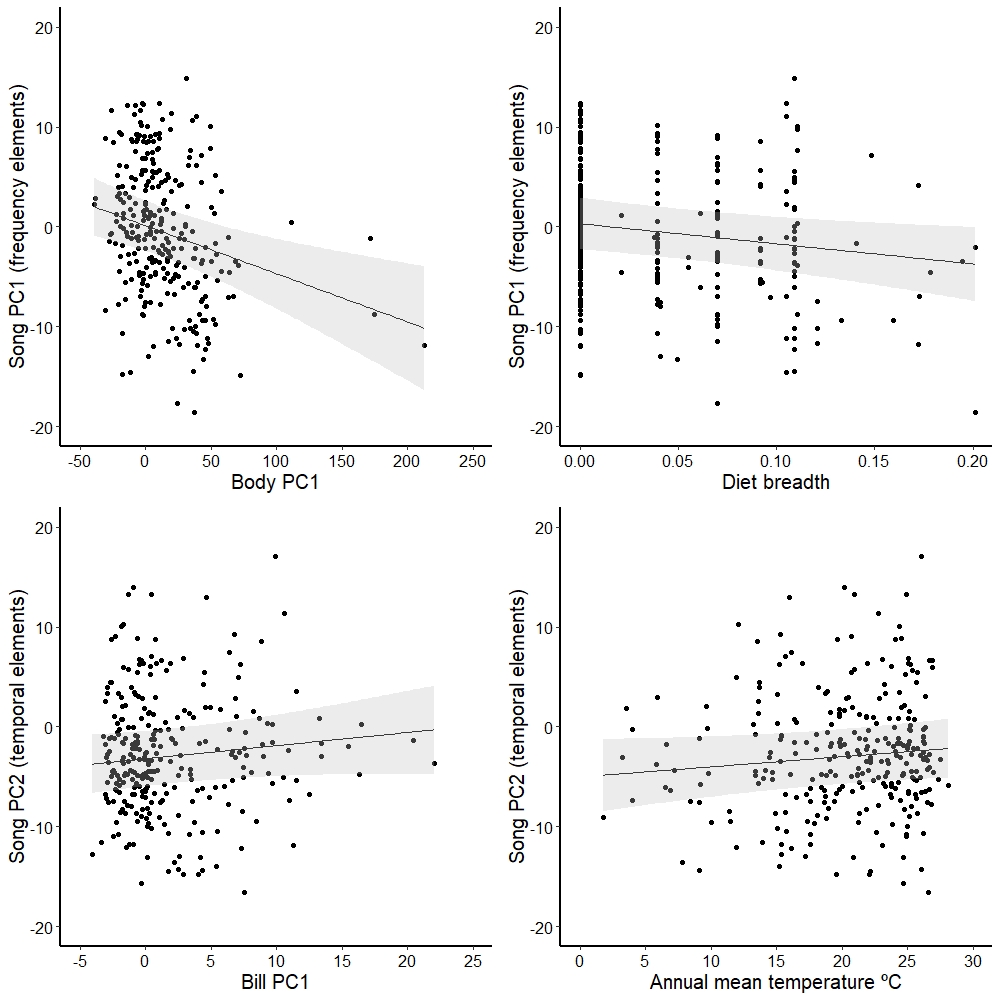
Fig. 2. Top panel: Consistent with the morphological adaptation hypothesis, Tyrannidae song frequency characteristics (Song PC1, 55.6%) were negatively associated with body size (Body PC1, 75.3%; left panel) and diet breadth (right panel). Bottom panel: The final model explaining song temporal components (Song PC2, 26.8%) included bill size (Bill PC1, 91%; left panel) and annual mean temperature (right panel), although the effects were not significant. Shaded area around regression line is SE. Higher song PC1 and PC2 scores represent higher frequency songs that are longer in duration, songs with more notes. Larger morphology PCs represent larger bodies and bills. Plotted data are corrected for phylogenetic relationships and other model covariates.
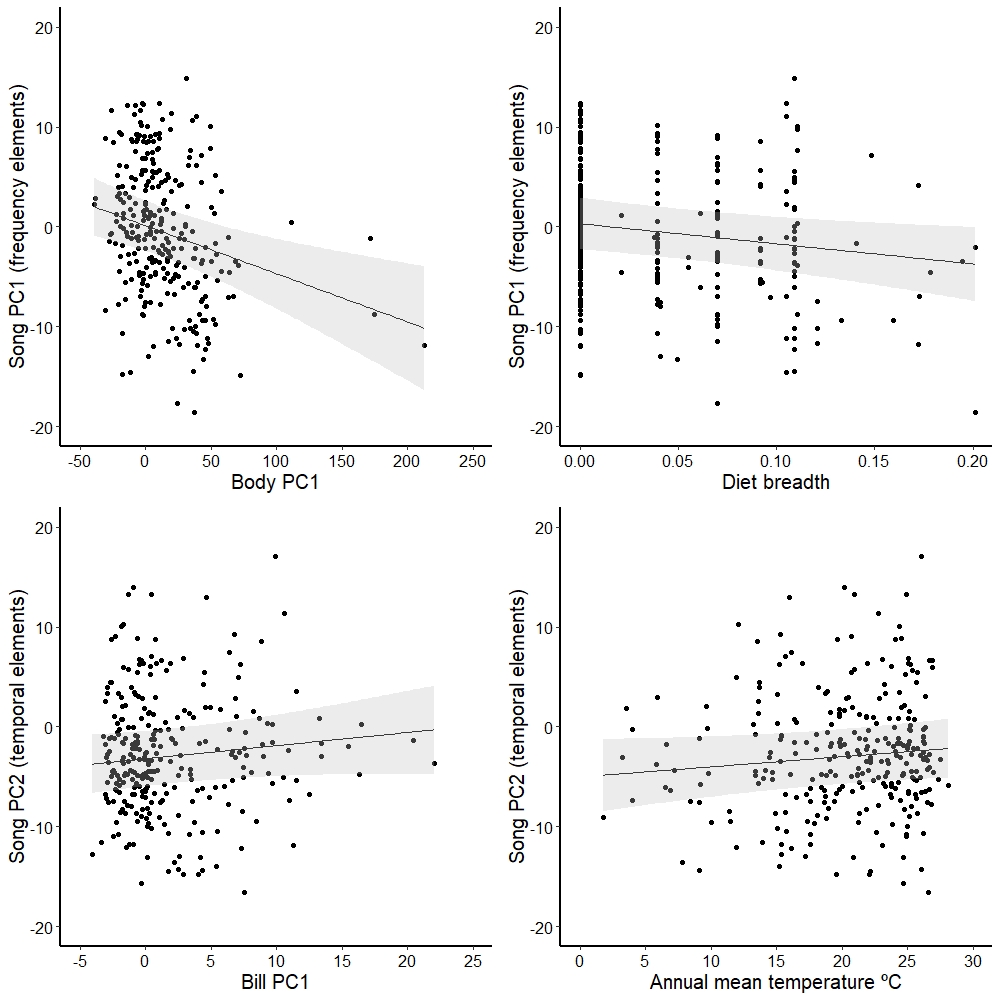
Fig. 3
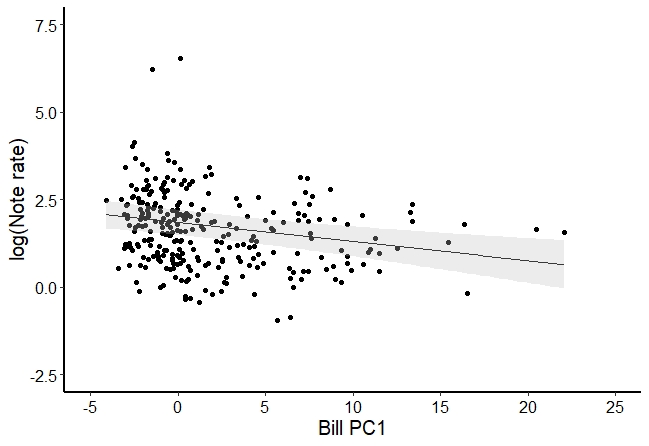
Fig. 3. In support of the morphological adaptation hypothesis, Tyrannidae species with heftier bills (Bill PC1, 91%) sang slower paced songs (note rate, log-transformed). Higher PC scores represent larger bills. Data are corrected for phylogenetic relationships and shaded area represents SE.
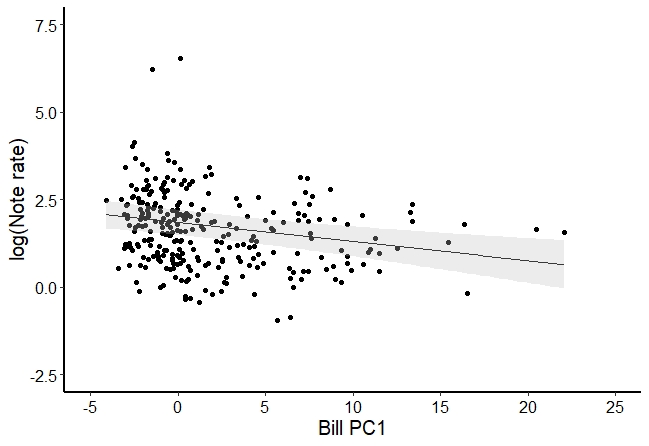
Fig. 4
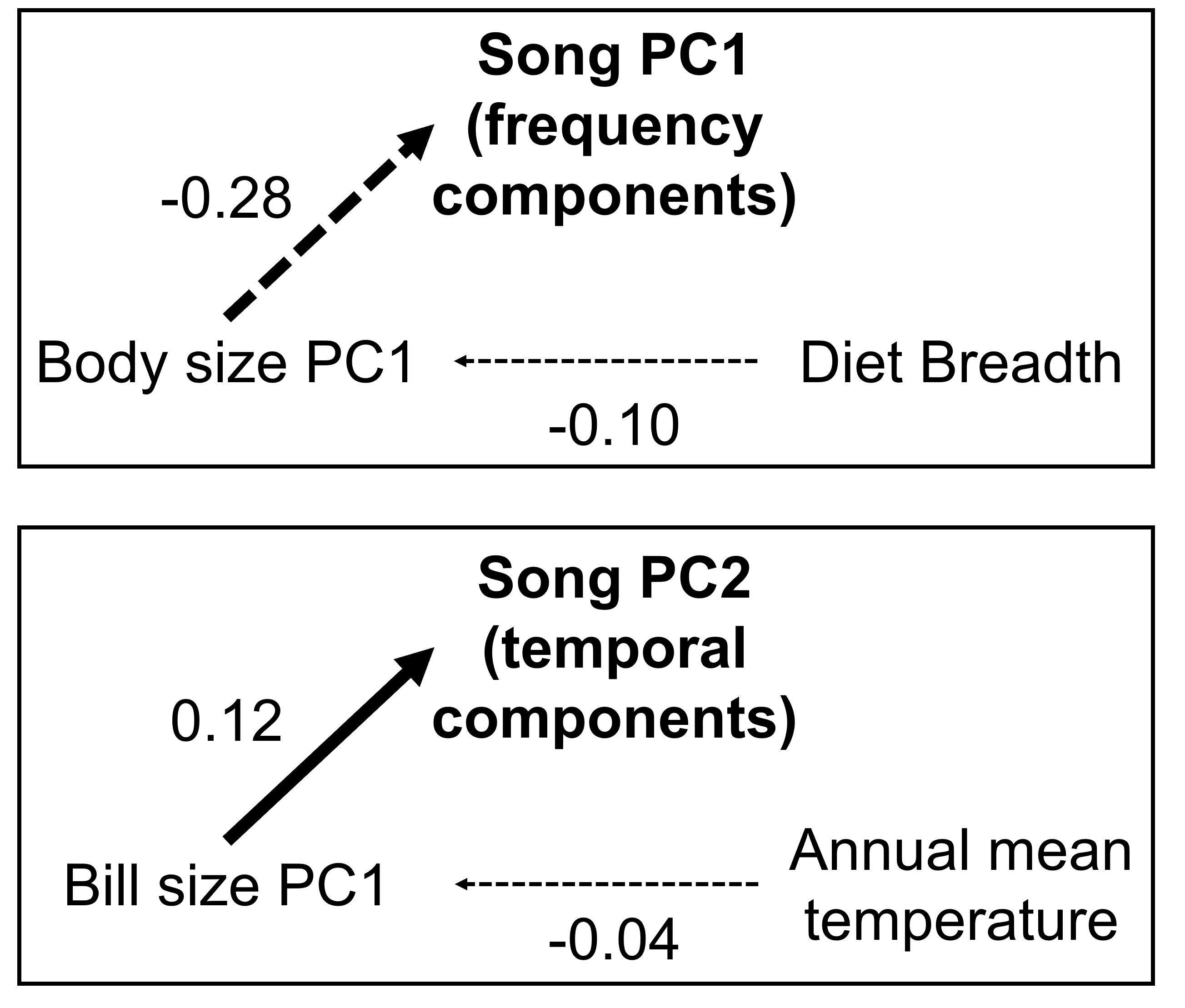
Fig. 4. Tyrannidae song evolution is constrained by morphology and covaries indirectly with ecology and climate. Schematic shows final phylogenetic generalized least square (PGLS)-informed phylogenetic path analysis models testing the relative importance of morphological, ecological, and climatic variables on Tyrannidae song evolution (song PC1, 55.6% [frequency] and PC2, 26.8% [temporal elements]). Solid arrows represent positive effects while dashed arrows represent negative effects, and arrows are scaled by their relative effect. Model coefficients are indicated adjacent to arrows.
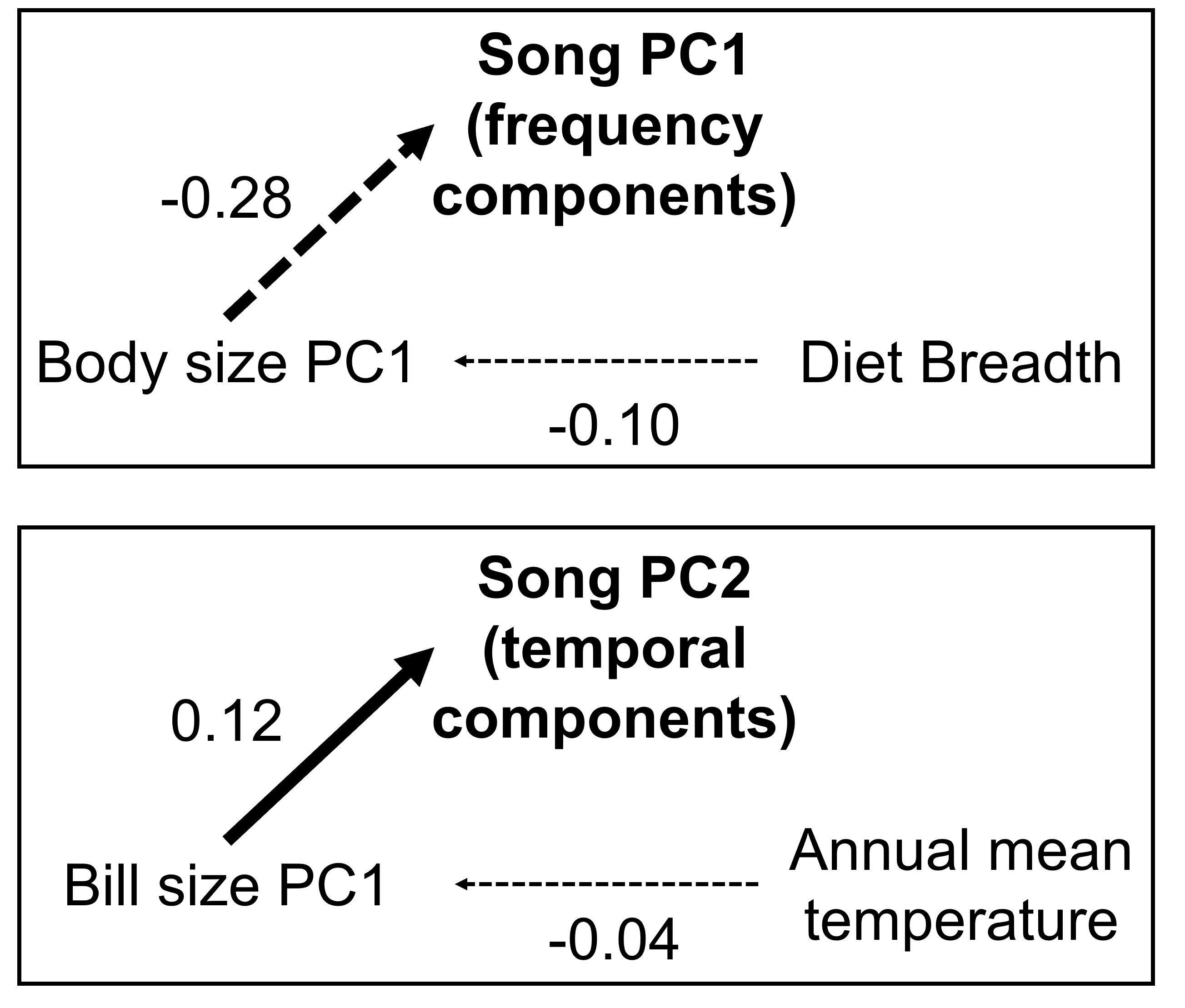
Table 1
Table 1. Full and final model selection results assessing the relationships between morphological, climatic, and ecological variables on Tyrannidae song PC1 (frequency elements) and song PC2 (temporal elements). Lambda (λ) indicates the phylogenetic signal of the response variable and was estimated using Pagel’s λ model of character evolution in a phylogenetic generalized least square analysis. PC scores were corrected for phylogenetic relationships. Note: K = number of model parameters; BIC = Bayesian Information Criterion score; AICc = Akaike’s Information Criterion score corrected for small sample sizes; ΔAICc = change between full and reduced model AICc; AICcWt = AICc weights, indicating the probability a model is the most parsimonious model; Cum.Wt = cumulative model weights; LL = log-likelihood.
Response | Model | Model Terms | K | BIC | AICc | ΔAICc | AICcWt | Cum.Wt | LL | λ |
|
PC1 | Final | Body PC1 + Diet Breadth | 3 | 1875.56 | 1857.57 | 0 | 0.92 | 0.92 | -923.68 | 0.17 | |
Full | Forest cover + Range Size + Bill PC1 + Body PC1 + Diet Breadth + Annual mean temp + Annual Precip | 8 | 1897.99 | 1862.38 | 4.81 | 0.08 | 1 | -920.79 | 0.20 | ||
PC2 | Final | Bill PC1 + Annual mean temp | 3 | 1812.81 | 1794.60 | 0 | 0.97 | 0.97 | -892.30 | 0.33 | |
Full | Forest cover + Range Size + Bill PC1 + Body PC1 + Diet Breadth + Annual mean temp + Annual Precip | 8 | 1837.16 | 1800.74 | 6.73 | 0.03 | 1 | -890.37 | 0.29 | ||
Table 2
Table 2. Akaike’s information criterion (AIC) selected model results from phylogenetic generalized least square models demonstrating the effects of morphology, climate, and ecology on song PC1 (frequency elements, 55.6%) and song PC2 (temporal elements, 26.8%). Also included are effect sizes (ϕ, 95% CI) for main effects. Note rate (number of song notes divided by song duration and log transformed) and Bill PC1 were tested separately because note rate was calculated independent of PCs and is hypothesized to covary with bill morphology. Significant results are shown in bold text. PC scores are corrected for phylogeny.
Response | Main Effect | Model df | Residual df | X² | p | ϕ | |||
Song PC1 (Frequency elements) |
Body PC1 | 1 | 279 | 11.10 | 0.0008 | 0.19 [0.06, 0.31] | |||
Diet Breadth | 1 | 279 | 5.39 | 0.02 | 0.12 [0, 0.25] | ||||
Song PC2 (Temporal elements) |
Bill PC1 | 1 | 279 | 2.26 | 0.13 | 0.07 [0, 0.2] | |||
Annual mean temperature | 1 | 279 | 2.38 | 0.12 | 0.07 [0, 0.2] | ||||
Note rate | Bill PC1 | 1 | 279 | 13.36 | 0.0003 | 0.21 [0.08-0.33] | |||
Table 3
Table 3. Tyrannidae genera showed mixed support for the species recognition hypothesis. Table summarizes phylogenetically controlled Mantel test results comparing Euclidean song distances and geographic proximity (km). Significant relationships based on alpha = 0.05 (p) are in bold, and in italics are Mantel correlation estimates (r) whose confidence intervals (CI) did not overlap 0 but p > 0.05. n refers to the number of species in the analysis.
Genus | r | p | Lower CI | Upper CI | n | ||||
All genera | -0.08 | 0.99 | -0.09 | -0.07 | 282 | ||||
Contopus | -0.09 | 0.69 | -0.35 | 0.18 | 11 | ||||
Elaenia | -0.03 | 0.54 | -0.27 | 0.21 | 12 | ||||
Empidonax | 0.04 | 0.26 | -0.15 | 0.23 | 15 | ||||
Hemitriccus | 0.06 | 0.25 | -0.15 | 0.26 | 14 | ||||
Myiarchus | -0.19 | 0.87 | -0.37 | 0.0 | 15 | ||||
Phyllomyias | 0.08 | 0.30 | -0.19 | 0.34 | 11 | ||||
Phylloscartes | 0.16 | 0.09 | -0.05 | 0.35 | 14 | ||||
Poecilotriccus | 0.38 | 0.10 | 0.10 | 0.61 | 10 | ||||
Tyrannus | 0.53 | 0.02 | 0.33 | 0.68 | 12 | ||||